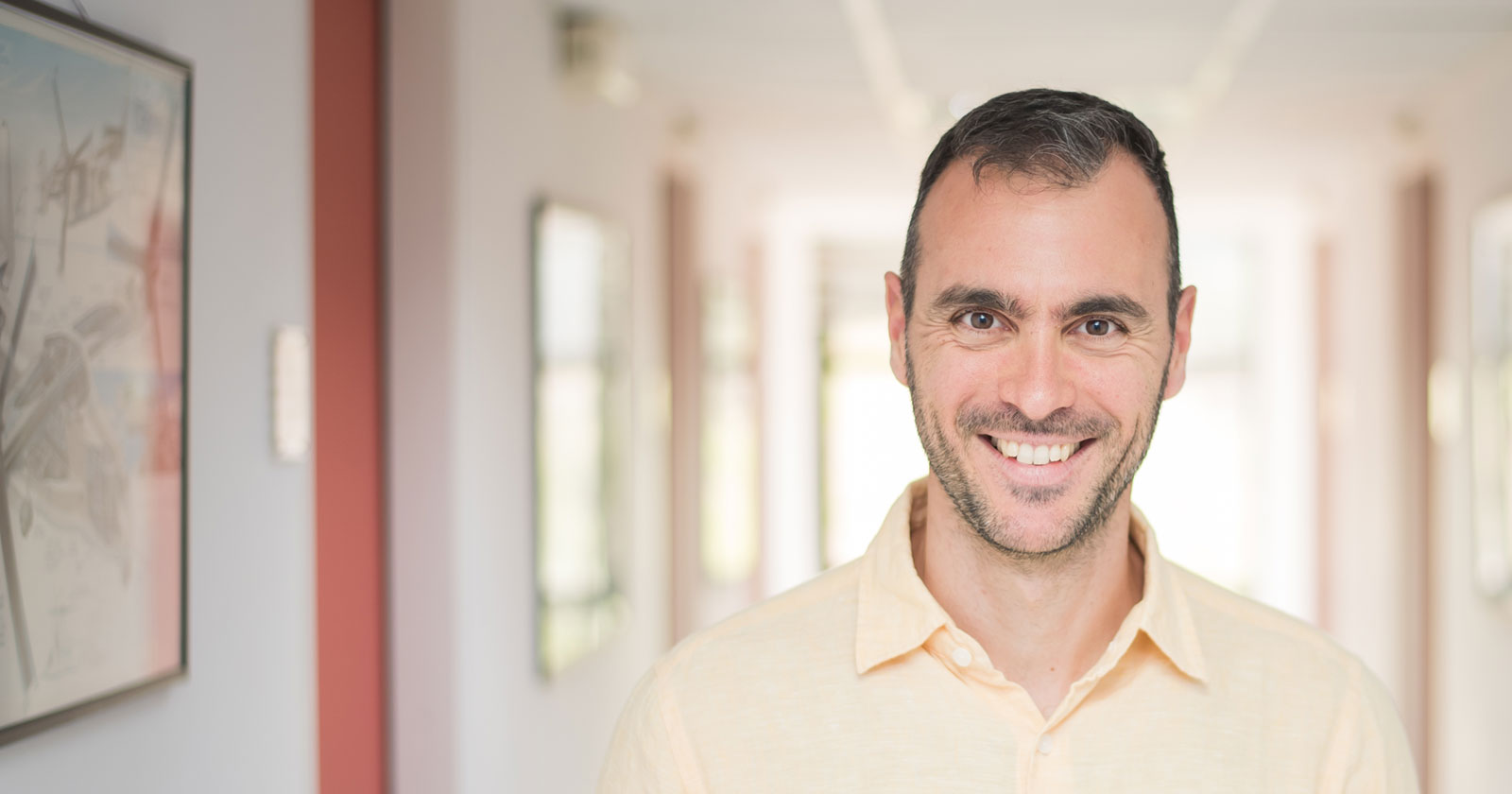
How Multiple Submissions May Be Distorting Real Outcomes in Machine Learning Challenges
SBV-IMPROVER organized the Metagenomics Diagnosis for IBD Machine Learning Challenge (MEDIC) aimed to investigate the diagnostic potential of metagenomics data to classify patients with Inflammatory Bowel Disease (IBD) and non-IBD subjects. The participants attempted to classify Ulcerative Colitis (UC) and Crohn’s Disease (CD) subjects with data obtained from non-invasive clinical samples. The challenge came with a prize pool of $12,000.
The aim was to find the best classification algorithm that can be used in diagnosing Inflammatory Bowel Disease with data obtained from non-invasive clinical samples. The basic question addressed by the participants was: Can you predict IBD status using metagenomics data?
More specifically, MEDIC aimed to verify that shotgun metagenomics sequencing data is sufficiently informative to allow for accurate classification of human subjects as:
IBD vs. non-IBD
UC vs. non-IBD
CD vs. non-IBD
UC vs. CD
With the analysis of the predictions submitted in the challenge, the goal was to answer the following scientific questions:
Which predictive computational approaches are the most accurate across the four 2-class problems described above?
What do the most discriminative metagenomic features tell us?
Are they rather based on taxonomy, functions/pathways and/or other types, e.g., k-mers?
Are they distinct between UC vs non-IBD and CD vs non-IBD or do they show commonalities?
The Machine Learning Sub-Challenge
There were two sub-challenges and JADBio participated in the second sub-challenge:
In the second sub-challenge (“MEDIC PROCESSED”), participants were provided with pre-calculated taxonomic and pathway abundances matrices derived from the raw data. This allowed data scientists with no access to metagenomics analysis pipelines to solve the Challenge, as well as to compare the performance of classification methods beyond the role of pre-processing steps. The organizers provided participants with shotgun metagenomic sequencing data as raw and processed data for predictor model training and testing.
The Results
JADBio’s automated machine learning findings were ranked in 4th place out of 13 participants. Although ranking might not be the ultimate goal compared to knowledge discovery our Senior Data Scientist at JADBio, Konstantinos Paraschakis, has a few thoughts on the total number of submissions and actual outcomes. He notes that “…this[4th place] doesn’t quite tell the whole story, in my opinion”. He argues that “There was a prize of 2,000 USD for each one of the top three teams in each sub-challenge and every participant could participate with multiple submissions. So, there were teams, obviously motivated by the prize, that participated with several submissions. And it was indeed those teams that made it to the top three. The winner team of the second sub-challenge, for example, submitted five prediction sets, the second winner eight, and the third winner… hold your breath… 32!!! So, if one team had sent 1000 submissions with completely randomly generated predictions, they would have most probably won the challenge with one of them”.

Konstantinos Paraschakis, Senior Data Scientist at JADBio
For those of you who are familiar with the JADBio algorithms, this is exactly what BBC-CV(Bootstrap Bias Correction) is trying to fix in the estimation of the best model’s performance. Below is a table of all participants of the second sub-challenge, along with the number of submissions by each one, their best rank, as well as their average rank. JADBio is the best performing team among teams with only one submission and has the best average ranking as well.
Team | Participations | Min Rank | Mean Rank |
CTLAB@ITMO | 5 | 1 | 26 |
mignon | 8 | 2 | 41 |
GiGi | 32 | 4 | 31 |
JADBio(Gnosis DA) | 1 | 6 | 6 |
JC | 1 | 7 | 7 |
UNIPI | 3 | 11 | 22 |
UNS(m) | 1 | 12 | 12 |
IBDClass | 1 | 13 | 13 |
DREAM | 1 | 15 | 15 |
CDS-Lab | 4 | 16 | 29 |
ArCHER-NIBIOHN | 1 | 43 | 43 |
ISDM Indonesia | 1 | 49 | 49 |
InSyBio | 1 | 60 | 60 |
To view full rankings go to the official website here.