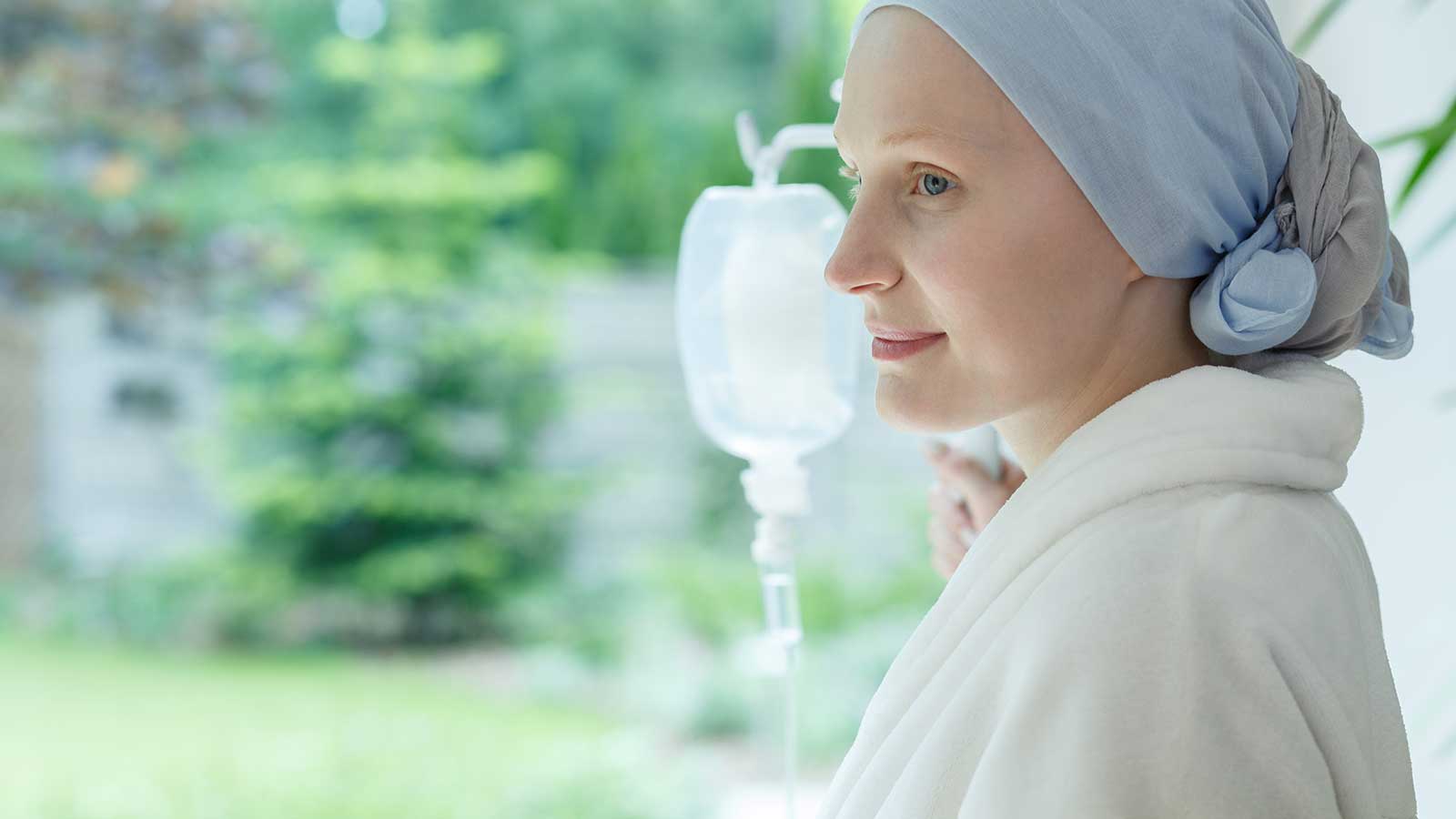
JADBio at the 9th Annual Health Informatics & Data Science Virtual Symposium
JADBio attended and presented virtually at Georgetown University‘s 9th Annual Health Informatics & Data Science Virtual Symposium. The symposium was held on October 2nd, 2020 with over 500 participants from all over the world. The Georgetown Innovation Center for Biomedical Informatics (ICBI) is a hub for cutting-edge research in biomedical informatics that applies data science methods and tools to advance Precision Medicine. Members of the ICBI engage in innovative research, workforce development, and impactful partnerships to advance data-inventive healthcare and life sciences domains, according to their website.
Within the scope of their work, Georgetown University held a health informatics data challenge regarding PrecissionFDA: Brain Cancer Predictive Modeling and Biomarker Discovery. Clinical investigators at Georgetown University are seeking to advance precision medicine techniques for the prognosis and treatment of brain tumors through the identification of novel multi-omics biomarkers. In support of this goal, precisionFDA and the Georgetown Lombardi Comprehensive Cancer Center and The Innovation Center for Biomedical Informatics at Georgetown University Medical Center (Georgetown-ICBI) are launching the Brain Cancer Predictive Modeling and Biomarker Discovery Challenge! This challenge will ask participants to develop machine learning and/or artificial intelligence models to identify biomarkers and predict patient outcomes using gene expression, DNA copy number, and clinical data.
The JADBio team won 3rd position and Konstantinos Paraschakis, Senior Data Scientist at JADBio, presented our autoML engine and its findings during the symposium. He analyzed brain cancer data, provided by ICBI using JADBio autoML engine. The analysis process is fully automated, requiring the minimum number of user decisions. He emphasized:
- how the engine thrives on small sample sizes and high dimensional feature spaces
- the discovery of statistically-equivalent feature subsets
- the best possible performance with the fewest possible features
- the accurate estimate of the model performance, and
- the interpretation-oriented output
Apart from the actual accurate predictive results that validate the finds of other analytical methodologies he also discussed what the future of the JADBio engine holds, describing how the team is working on incorporating new algorithms and estimation protocols to scale up to millions of features and samples. They also want to broaden the input array to include images as features (soon to be released), incorporate batch correction, and finally allow access through a public API.
Watch Konstantinos Paraschakis’ full presentation on YouTube