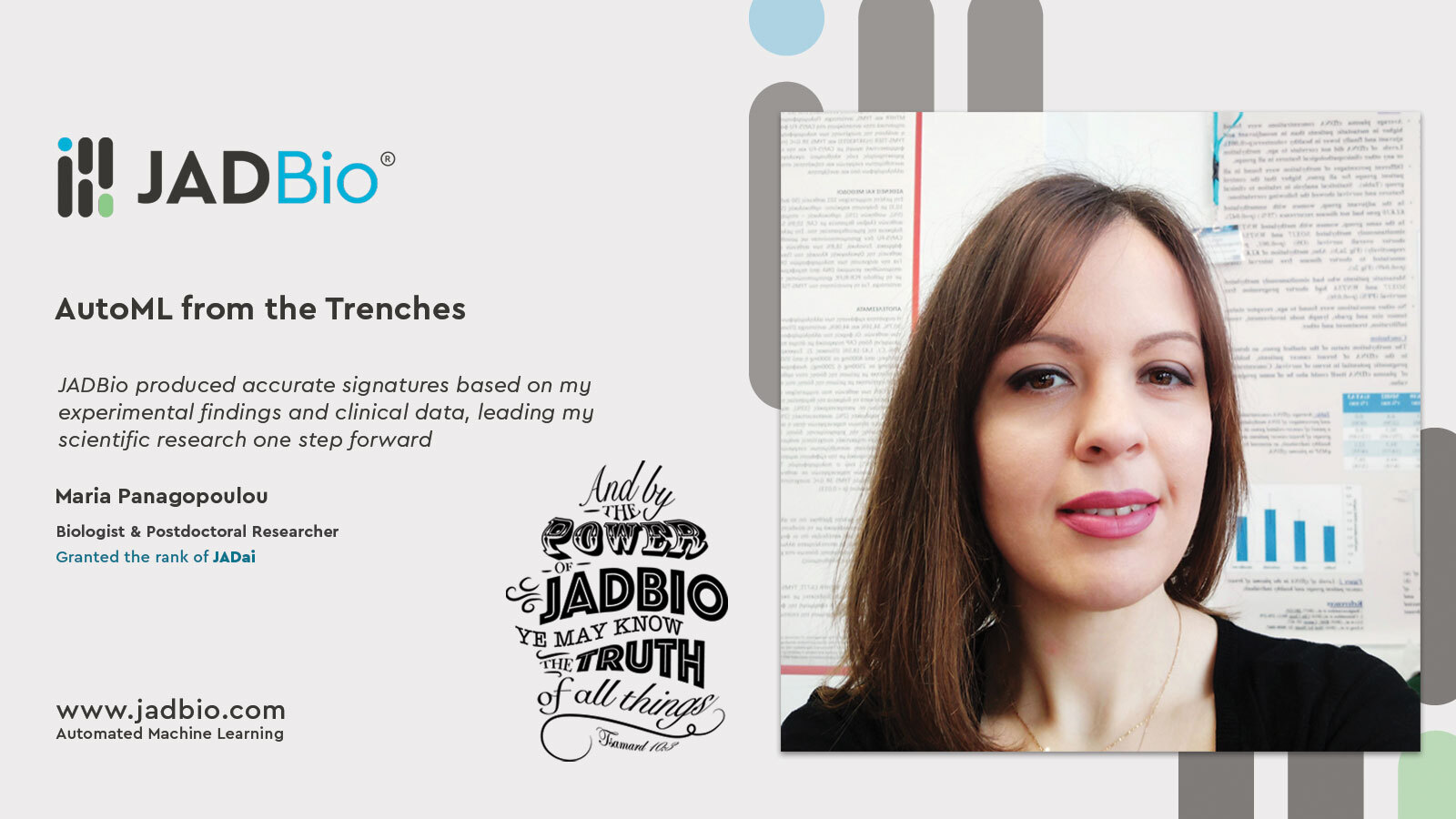
Maria Panagopoulou – JADBio auto ML from the Trenches
Maria Panagopoulou is a biologist and postdoctoral researcher in the field of cancer and biomarker research in the research group of Professor Dr. Aikaterini Chatzaki (Lab of Pharmacology, Dept of Medicine, DUTH). Talking about her experience with JADBio auto ML, she said she first used the platform for analyzing her Ph.D. data. JADBio’s automated machine learning tool led her scientific research one step forward by producing accurate signatures based on her experimental findings and clinical data. Her research, “Circulating cell-free DNA in breast cancer: size profiling, levels, and methylation patterns lead to prognostic and predictive classifiers,” was published in the highest impact factor journal Oncogene.
In our question about why she would recommend JADBio, Maria replied, “I would definitely recommend JADBio to colleagues as in an easy way can exploit their research findings and get reliable signatures. In general, JADBio provides predictive models by employing standard, best practices, and state-of-the-art statistical and machine learning methods. JADBio automatically produces predictive models either for a discrete (classification), or a continuous (regression), or a time-to-event (survival analysis) outcome and highlights novel biomarkers, offering a great advantage in biomedical research.”
Abstract
Blood circulating cell-free DNA (ccfDNA) is a suggested biosource of valuable clinical information for cancer, meeting the need for a minimally-invasive advancement in the route of precision medicine. This paper evaluated the prognostic and predictive potential of ccfDNA parameters in early and advanced breast cancer. Groups consisted of 150 and 16 breast cancer patients under adjuvant and neoadjuvant therapy, respectively, 34 patients with metastatic disease and 35 healthy volunteers. Direct quantification of ccfDNA in plasma revealed elevated concentrations correlated to the incidence of death, shorter PFS, and non-response to pharmacotherapy in the metastatic but not in the other groups. The methylation status of a panel of cancer-related genes chosen based on previous expression and epigenetic data (KLK10, SOX17, WNT5A, MSH2, GATA3) was assessed by quantitative methylation-specific PCR. All but the GATA3 gene was more frequently methylated in all the patient groups than in healthy individuals (all p < 0.05). The methylation of WNT5A was statistically significantly correlated to greater tumor size and poor prognosis characteristics and in advanced-stage disease with shorter OS. In the metastatic group, also SOX17 methylation was significantly correlated to the incidence of death, shorter PFS, and OS. KLK10 methylation was significantly correlated to unfavorable clinicopathological characteristics and relapse, whereas in the adjuvant group to shorter DFI. Methylation of at least 3 or 4 genes was significantly correlated to the shorter OS and no pharmacotherapy response, respectively. Classification analysis by a fully automated machine learning software produced a single-parametric linear model using ccfDNA plasma concentration values, with great discriminating power to predict response to chemotherapy (AUC 0.803, 95% CI [0.606, 1.000]) in the metastatic group. Two more multi-parametric signatures were produced for the metastatic group, predicting survival and disease outcome. Finally, a multiple logistic regression model was constructed, discriminating between patient groups and healthy individuals. Overall, ccfDNA emerged as a highly potent predictive classifier in metastatic breast cancer. Upon prospective clinical evaluation, all the signatures produced could aid accurate prognosis.