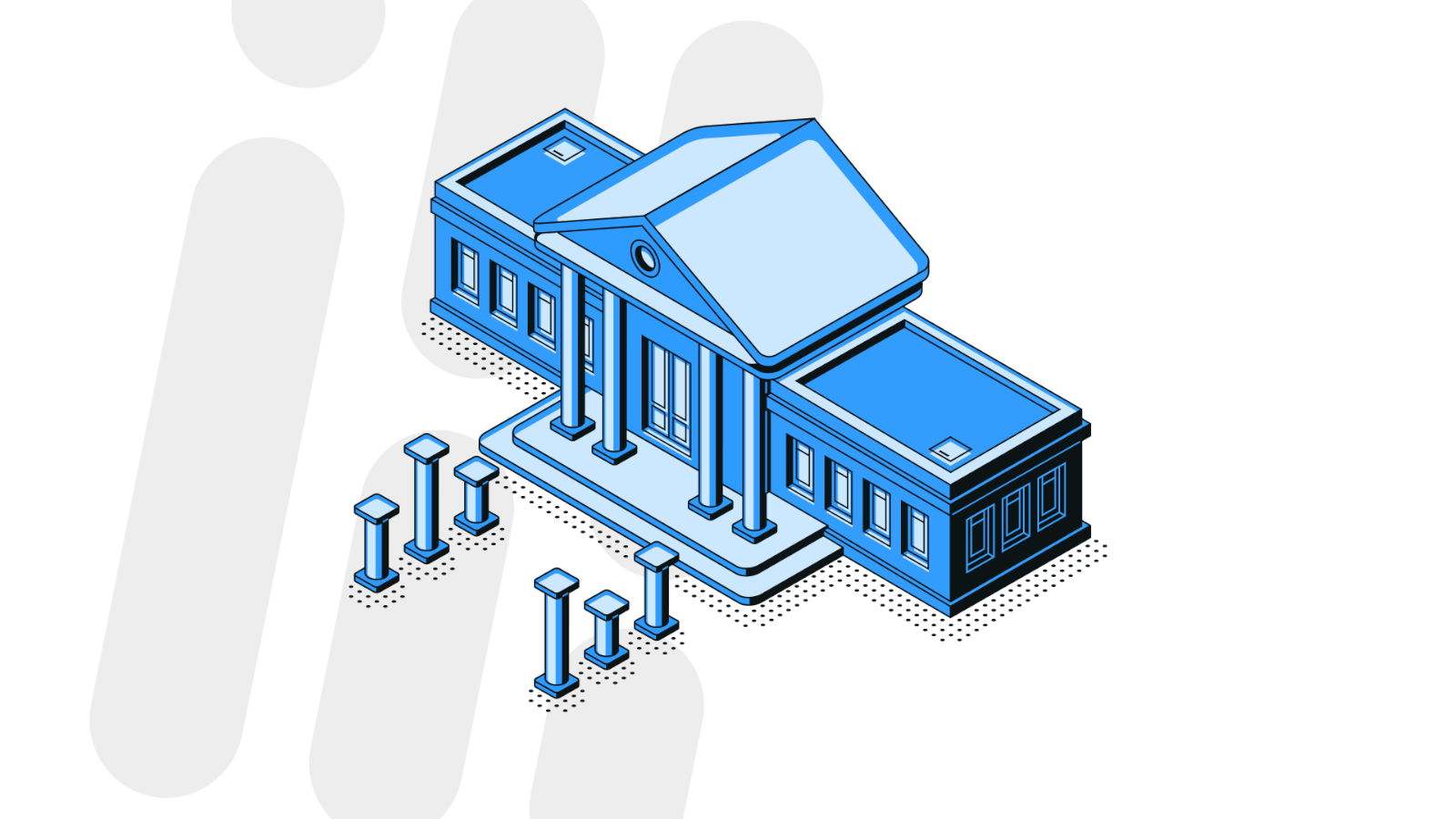
An AutoML architecture for the accelerated prediction of Metal-Organic Frameworks
Published in: Microporous and Mesoporous Materials, Volume 300, 110160, 15 June 2020
Authors
Ioannis Tsamardinos
George S. Fanourgakis, Elissavet Greasidou, Emmanuel Klontzas, Konstantinos Gkagkas, George E.Froudakis
Abstract
Due to their exceptional host-guest properties, Metal-Organic Frameworks (MOFs) are promising materials for storage of various gases with environmental and technological interest. Molecular modeling and simulations are invaluable tools, extensively used over the last two decades for the study of various properties of MOFs. In particular, Monte Carlo simulation techniques have been employed for the study of the gas uptake capacity of several MOFs at a wide range of different thermodynamic conditions. Despite the accurate predictions of molecular simulations, the accurate characterization and the high-throughput screening of the enormous number of MOFs that can be potentially synthesized by combining various structural building blocks is beyond present computer capabilities. In this work, we propose and demonstrate the use of an alternative approach, namely one based on an Automated Machine Learning (AutoML) architecture that is capable of training machine learning and statistical predictive models for MOFs’ chemical properties and estimates their predictive performance with confidence intervals. The architecture tries numerous combinations of different machine learning (ML) algorithms, tunes their hyper-parameters, and conservatively estimates the performance of the final model. We demonstrate that it correctly estimates performance even with few samples (<100) and that it provides improved predictions over trying a single standard method, like Random Forests. The AutoML pipeline democratizes ML to non-expert material-science practitioners that may not know which algorithms to use on a given problem, how to tune them, and how to correctly estimate their predictive performance, dramatically improving productivity and avoiding common analysis pitfalls. A demonstration on the prediction of the carbon dioxide and methane uptake at various thermodynamic conditions is used as a showcase sharable:
Keywords
Automated machine learning, Metal-organic frameworks, Carbon dioxide, Methane, Environment, Energy