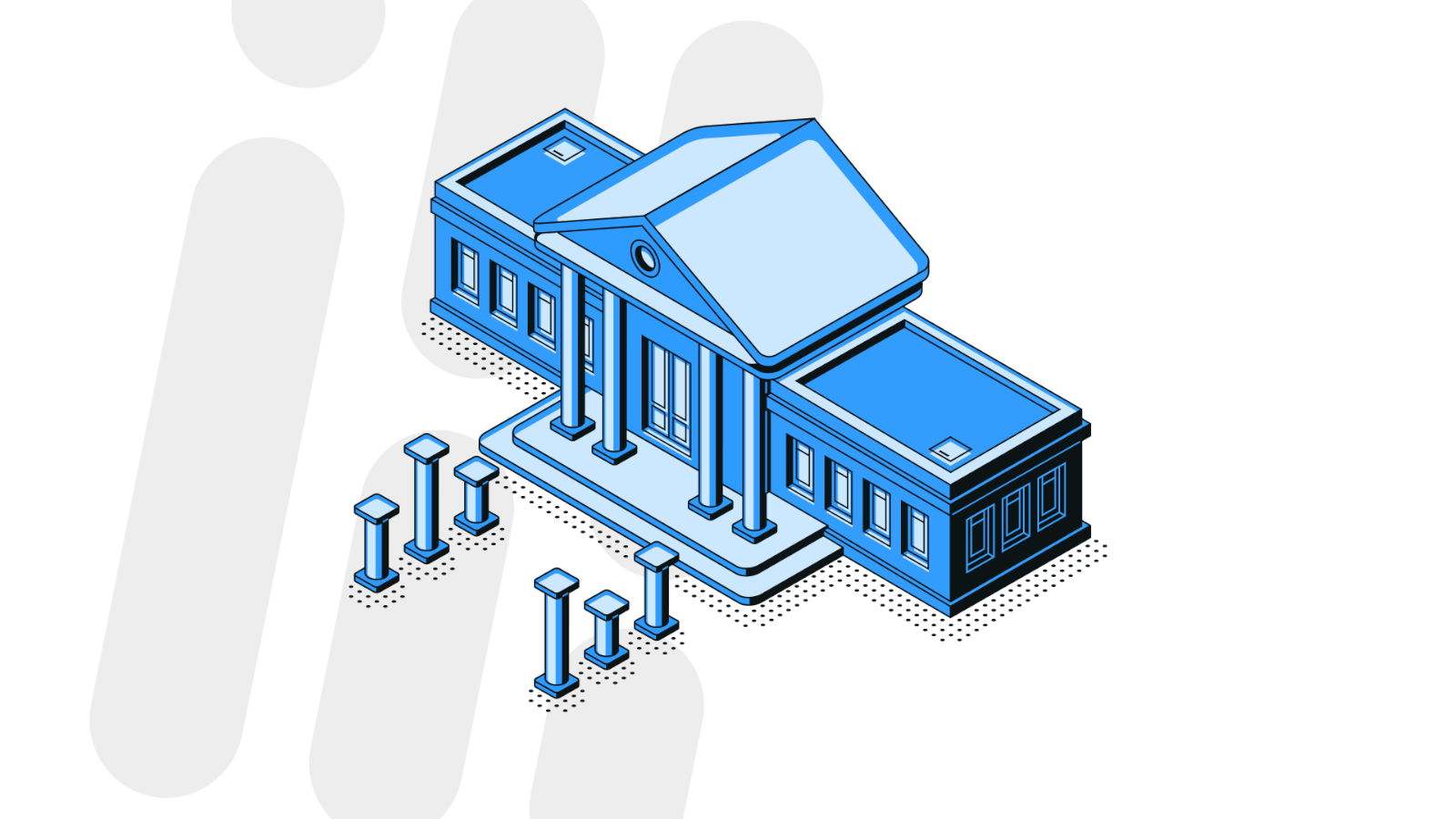
Applicability of an Automated Model and Parameter Selection
Published in: JMIR Medical Informatics, 8(7), e17119, 16 April 2020
Authors
Ioannis Tsamardinos
Karen-Inge Karstoft, Kasper Eskelund, Søren Bo Andersen, Lars Ravnborg Nissen
Abstract
Background:
Posttraumatic Stress Disorder (PTSD) is a relatively common consequence of deployment to war zones. Early post-deployment screening with the aim of identifying those at risk for PTSD in the years following deployment will help target interventions to those in need but have so far proved unsuccessful.
Objective:
To test the ability of ML-based prediction models to predict screening-level PTSD 2.5 years and 6.5 years after deployment. Further, we test if models trained in one cohort can be transferred to other cohorts.
Methods:
Automated machine learning (AutoML) applied to data collected routinely 6-8 months after return from deployment on three different cohorts of Danish soldiers deployed to Afghanistan in 2009 (Cohort 1, N=287/261), 2010 (Cohort 2, N=352) and 2013 (Cohort 3, N=232).
Results:
For 2.5-year screening-level PTSD, we find that a Random Forest model provides the highest accuracy as measured by area under the ROC-curve (AUC=0.77, CI=0.71-0.83), which is also the case for predicting 6.5-year screening-level PTSD (AUC=0.78, CI=0.73-0.83). Military rank, hypervigilance, and level of PTSD-symptoms are consistent predictors.
Conclusions:
The validated models can be readily implemented in future deployment cohorts in the Danish Defense with the aim of targeting post-deployment support interventions to those at the highest risk for developing PTSD, provided the cohorts are deployed on similar missions.