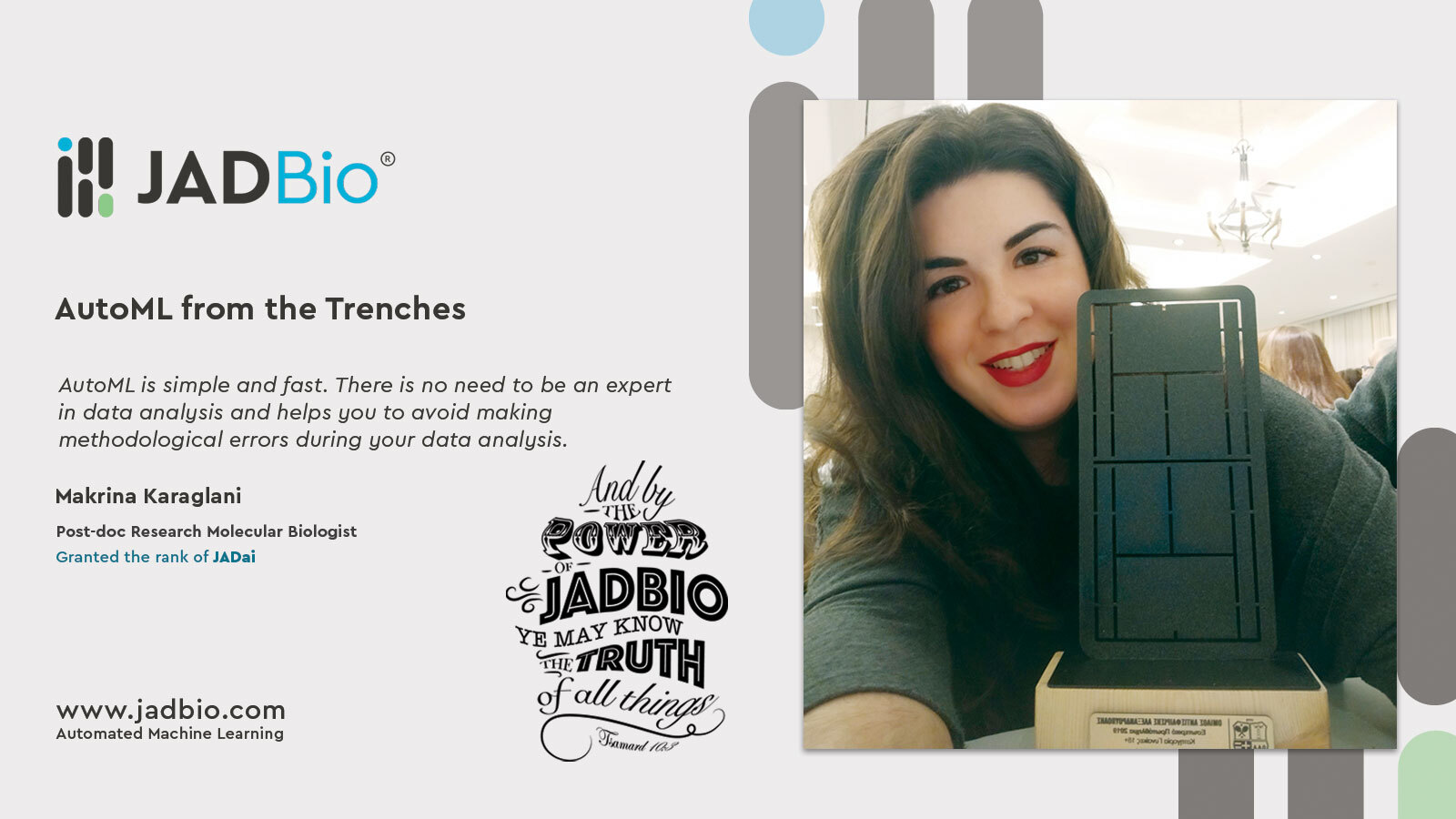
Makrina Karaglani – JADBio autoML from the Trenches
Makrina Karaglani is a post-doc research molecular biologist working on circulating DNA methylation biomarkers for the early diagnosis of diseases such as diabetes and breast cancer. Talking about machine learning and autoML, the latter in her opinion will facilitate the medical decision-making process. Given that Makrina is a beginner in data analysis with no coding skills what she sees as key advantages in autoML is that is simple and fast. “There is no need to be an expert in data analysis and helps you to avoid making methodological errors during your data analysis. You are sure that you analyzed your data correctly.”
During her research, Accurate Blood-Based Diagnostic Biosignatures for Alzheimer’s Disease via Automated Machine Learning, she’s been working with the JADbio auto ML platform and analyzed several datasets. What she experienced is JADBio making her life easier by analyzing different omic datasets consisting of thousands of hundreds of features in just a few minutes without any prior knowledge in machine learning.
“I would suggest JADBio to colleagues because it is a user-friendly platform that offers a variety of machine learning tasks and provides a lot of visualizations of our results.”
Accurate Blood-Based Diagnostic Biosignatures for Alzheimer’s Disease via Automated Machine Learning
Abstract
Alzheimer’s disease (AD) is the most common form of neurodegenerative dementia and its timely diagnosis remains a major challenge in biomarker discovery. In the present study, we analyzed publicly available high-throughput low-sample -omics datasets from studies in AD blood, by the AutoML technology Just Add Data Bio (JADBIO), to construct accurate predictive models for use as diagnostic biosignatures. Considering data from AD patients and age-sex matched cognitively healthy individuals, we produced three best performing diagnostic biosignatures specific for the presence of AD: A. A 506-feature transcriptomic dataset from 48 AD and 22 controls led to a miRNA-based biosignature via Support Vector Machines with three miRNA predictors (AUC 0.975 (0.906, 1.000)), B. A 38,327-feature transcriptomic dataset from 134 AD and 100 controls led to six mRNA-based statistically equivalent signatures via Classification Random Forests with 25 mRNA predictors (AUC 0.846 (0.778, 0.905)) and C. A 9483-feature proteomic dataset from 25 AD and 37 controls led to a protein-based biosignature via Ridge Logistic Regression with seven protein predictors (AUC 0.921 (0.849, 0.972)). These performance metrics were also validated through the JADBIO pipeline confirming stability. In conclusion, using the automated machine learning tool JADBIO, we produced accurate predictive biosignatures extrapolating available low sample -omics data. These results offer options for minimally invasive blood-based diagnostic tests for AD, awaiting clinical validation based on respective laboratory assays. They also highlight the value of AutoML in biomarker discovery.