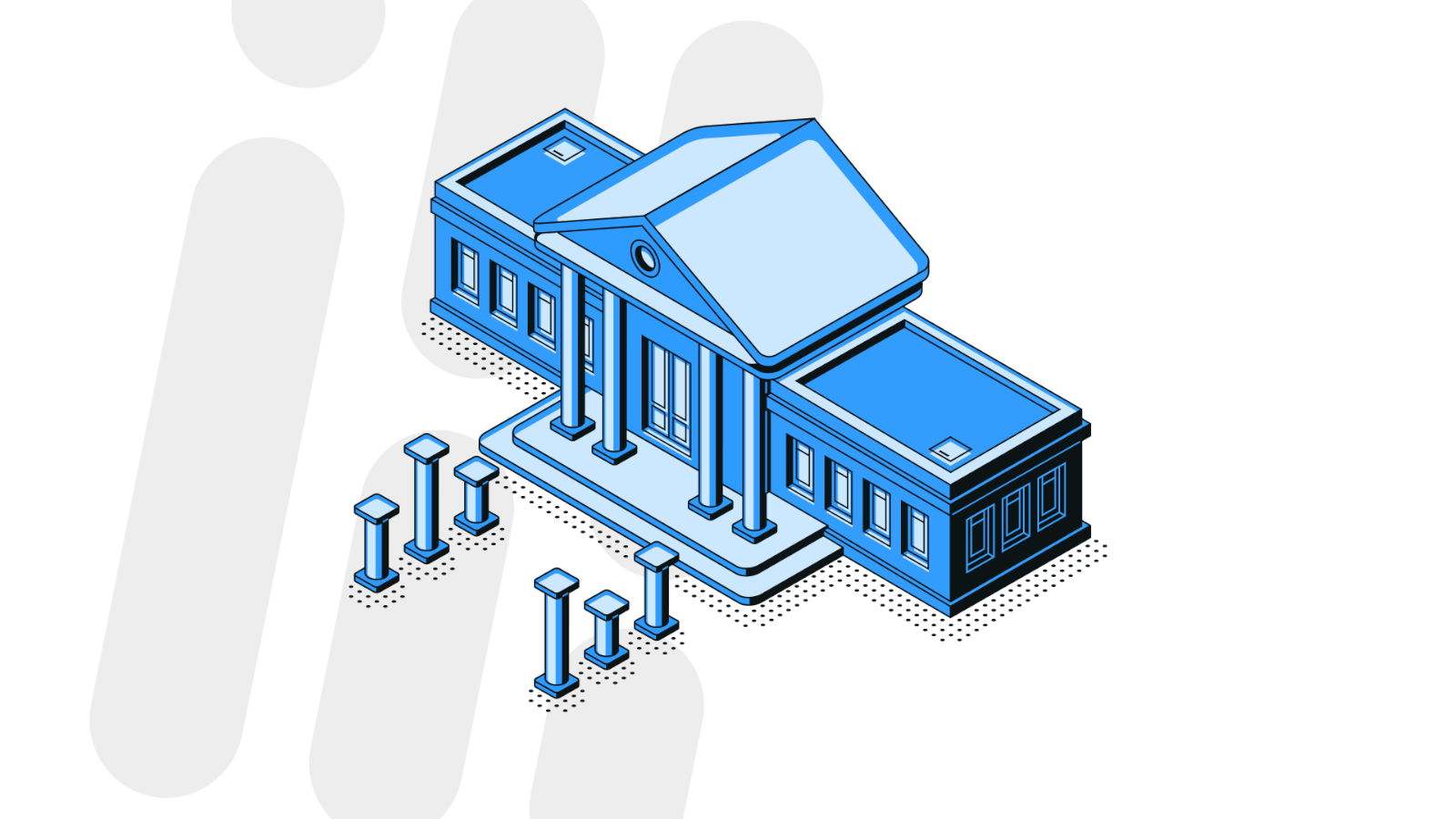
Putting the Human Back in the AutoML Loop
Published in: Workshop Proceedings of the EDBT/ICDT 2020 Joint Conference (March 30-April 2, 2020, Copenhagen, Denmark)
Authors
Ioannis Tsamardinos
Iordanis Xanthopoulos, Vassilis Christophides, Eric Simon, Alejandro Salinger
Abstract
Automated Machine Learning (AutoML) is a rapidly rising subfield of Machine Learning. AutoML aims to fully automate the machine learning process end-to-end, democratizing Machine Learning to non-experts and drastically increasing the productivity of expert analysts. So far, most comparisons of AutoML systems focus on quantitative criteria such as predictive performance and execution time. In this paper, we examine AutoML services for predictive modeling tasks from a user’s perspective, going beyond predictive performance. We present a wide palette of criteria and dimensions on which to evaluate and compare these services as a user. This qualitative comparative methodology is applied on seven AutoML systems, namely Auger.AI, BigML, H2O’s Driverless AI, Darwin, Just Add Data Bio, RapidMiner, and Watson. The comparison indicates the strengths and weaknesses of each service, the needs that it covers, the segment of users that is most appropriate for, and the possibilities for improvements.
Keywords
AutoML, machine learning services, qualitative evaluation