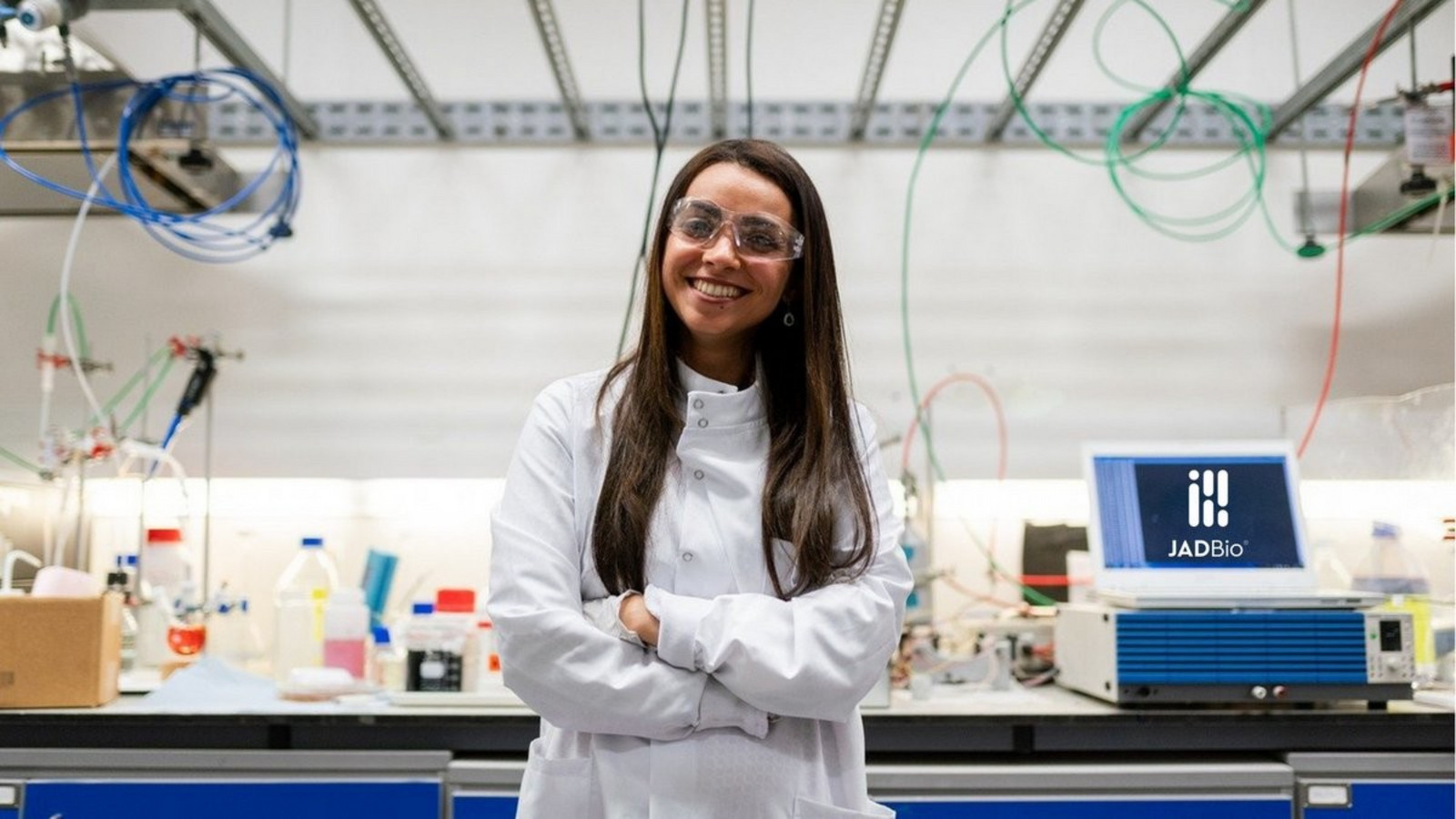
The future of medical treatment lies where AutoML and life scientists meet
First published on ITProPortal, by Benedict Timmerman –Senior IT Experience Analyst.
AutoML allows life scientists to be life scientists, doing what they do best, analyzing the final information, and making educated connections between data and disease. Alzheimer’s Disease (AD) is the fifth leading cause of death for those aged 65 plus. There is currently no definitive diagnosis short of autopsy, and scientists are racing the clock against the arrival of the oldest average population in history. With this push comes an abundance of study data, and there just aren’t enough data scientists to keep up with analyzing it all. Enter automated machine learning (autoML), which solves this problem by automating the machine learning process end-to-end.
What is AutoML?
More than 70 years after the invention of the first computer, these are no longer just objects of wonder. And, they’ve become extremely intelligent. Machine learning is the study of computer algorithms that improve automatically through experience. Sounds pretty smart, right? These algorithms are patterns that can provide critical insight into diseases such as Alzheimer’s. However, in order to be useful, you need a large set of them doing many different sophisticated processes each, running thousands of combinations on your data, choosing the best feature sets to give prominence to, tweaking for best predictive performance…and all that has to be done by a scientist who understands what the outcome means. It’s sort of combining two different disciplines into one; biology and computer science into one. While such a discipline does exist, it’s your bioinformatician, the whole process of building machine learning models is no easy feat. It usually takes a lot of time, often months, and programming knowledge to choose the right algorithms, tweak for hyperparameters, and in the end, create a predictive model that gives you the best performing results for your problem.
This is where AutoML comes in to automate this process, save time for the scientist, and allow the application of sophisticated algorithms to real-world problems. As the cost to store Big Data goes down, it becomes more accessible, and as global emergencies such as the Covid-19 pandemic accentuate how necessary it is to have it translated in real-time, the appetite for this to be made simpler grows. AutoML has the power to bring crucial data mining tools such as statistical modeling, pattern recognition, and analyses replicability to life-scientists’ fingertips.
AutoML Means Doing Data Analysis Faster
As AutoML becomes smarter, researchers will be able to isolate and identify such new biomarkers for diseases like Alzheimer’s in a more efficient manner, enabling diagnostics and prognoses that are less intrusive and more cost-effective.
JADBio, an L.A. startup, is an AutoML platform created by machine learning scientists for life scientists. It aims to accelerate the progress toward a cure for AD, infectious diseases, and numerous other maladies that cost countless lives every year. The platform allows anyone, ranging from a data scientist with deep knowledge of ML to a clinician with no expertise in coding, to upload their data —for example, a data set of 15 patients with a history of Alzheimer’s in their families— and a very large feature set, including genetic and other biomedical information. They can select multiple feature sets, tweak for overfitting, and produce a predictive model, assessing the possibility of Alzheimer’s in a fraction of the time it would take them to do it manually. AutoML makes data analysis easy for non-experts and efficient for the experts.
JADBio and Alzheimer’s
The Ubiquitous Use of AutoML in MedicineIn Alzheimer’s disease, brain changes that occur before the onset of symptoms are currently only detectable through expensive tests like positron emission tomography (PET) scans and invasive ones like spinal fluid collection (CSF). Those at high risk for AD would have to want to know pretty badly in order to undergo one of these.
It was announced, during last year’s Alzheimer’s Association International Conference (AAIC 2020), by an international team of researchers that they identified a highly accurate, blood-based biomarker for the detection of Alzheimer’s disease by measuring levels of p-tau217 in blood and validated the finding in multiple, diverse populations. A biomarker is a measurable indicator of the severity or presence of a disease. Once verified, this biomarker – and possibly others yet to be discovered – could open the door to a simple blood test with the ability to determine risk.
Because most medical professionals are not computer scientists, technology like AutoML is designed to provide these predictive analytics with minimal human intervention. In this way, it requires no greater expertise than you would need to open up a spreadsheet. Then the medical practitioners are free to interpret the data for the betterment of society. A research team led by Makrina Karaglani, a post-doc research molecular biologist, put the JADBio platform to the test with three different AD studies. Karaglani, who is a beginner in data analyses with no coding skills to speak of, reported that the greatest advantage of AutoML is that it is fast and simple.
“There is no need to be an expert in data analysis and helps you to avoid making methodological errors during your data analysis. You are sure that you analyzed your data correctly”
Makrina Karaglani, Postdoctoral Researcher, Molecular Biologist
Using publicly available repositories of blood taken from both AD patients and healthy individuals, Karaglani and her colleagues reprocessed seven high-throughput, low-sample -omics datasets and produced three accurate predictive models for diagnostic biosignatures for the presence of AD. They went on to use the extensive JADBio pipeline to confirm the stability of those biomarkers.
“We show that Alzheimer’s can be accurately diagnosed by measuring just a few biomarkers in the blood. This could one day lead to a practical and molecular Alzheimer’s diagnostic test. In addition, these biomarkers could provide insight into the biological mechanisms of the disease”
Ioannis Tsamardinos, Professor in AI and CEO at JADBio.
The JADBio technology also has the intuitive capability of allowing scientists to cross-reference with other artificial intelligence (AI) platforms such as the Human Gene database tool, GeneCards, where the team was able to search for correlations in proteins mRNAs and miRNAs common to AD.
The ubiquitous use of AutoML in medicine
In a 2019 feasibility study funded by the National Institute for Health Research and Moorfields Eye Charity, researchers set out to evaluate the value of AutoML software, and more specifically Google’s Cloud AutoML, when used by healthcare professionals with no coding or deep learning expertise to develop medical image diagnostic classifiers.
The results indicated that the value was there:
“The two models trained to distinguish multiple classification tasks showed high diagnostic properties and discriminative performance,” stated the researchers in the Lancet Digital Health article.
We are currently living in the midst of another perfect example of a major push for scientific information that AutoML could make a lot easier to manage.
Dr. Kenji Ikemura, a resident physician at Einstein Medical Center in the Bronx, NY, led a research team in a study to determine if AutoML could help them make the impossible decision as to which Covid-19 patients received limited life-saving resources. The team developed and compared multiple ML models that best predicted the odds of patient survival.
With the use of AutoML, they developed high-performing models that predicted patient mortality from Covid-19 and discovered the important biomarkers correlated with mortality. “This ML model can be used as a decision-supporting tool for medical practitioners to triage Covid-19 infected patients efficiently. From our literature review, this will be the largest Covid-19 patient cohort to train ML models and the first to utilize AutoML”. They went as far as to create a Covid-19 survival calculator based on their study.
The future of AutoML in biomedical analysis
Professor Ioannis Tsamardinos says that AutoML can shine a new light on historical information that could make a difference today in diagnostic efforts such as the ones mentioned here.
“Old” data have been used in prior publications using the analysis methods that were available at the time of publishing. In addition, it is impossible for any single research group to optimally apply all possible methods of analysis. This means that a lot of knowledge is hidden inside these Old data that has been untapped and could be extracted with modern AutoML technologies,” he said.
“I think AutoML for biomedical analysis will grow in scope, in terms of the type of data it can analyze, for example, images, signals, free text, and many others and their combination, the type of analyses – automated clustering or even causal analysis. It will also put more and more emphasis on explainability, interpretability of results, and fair analysis to exclude possible discrimination by the predictive models,” he added.
So autoML allows life scientists to be life scientists, doing what they do best, analyzing the final information, and making educated connections between data and disease. Studies such as these show that the answer to the diagnostic dilemma of “too much information” may be found where autoML and biomedical analysis meet.
JADBio makes it easy and affordable for health-data analysts and life science professionals to use data science to discover knowledge while reducing time and effort by combining a robust end-to-end machine learning platform with a wealth of capabilities.
Grab a FREE Basic plan here – What are you waiting for?